Accuracy, disease predictability related to precision dairy technology evaluated
Study looks at technology's ability to detect health events, machine-learning's ability to improve disease detection and incorporation of cow history with behavior changes as it relates to improved disease detection.
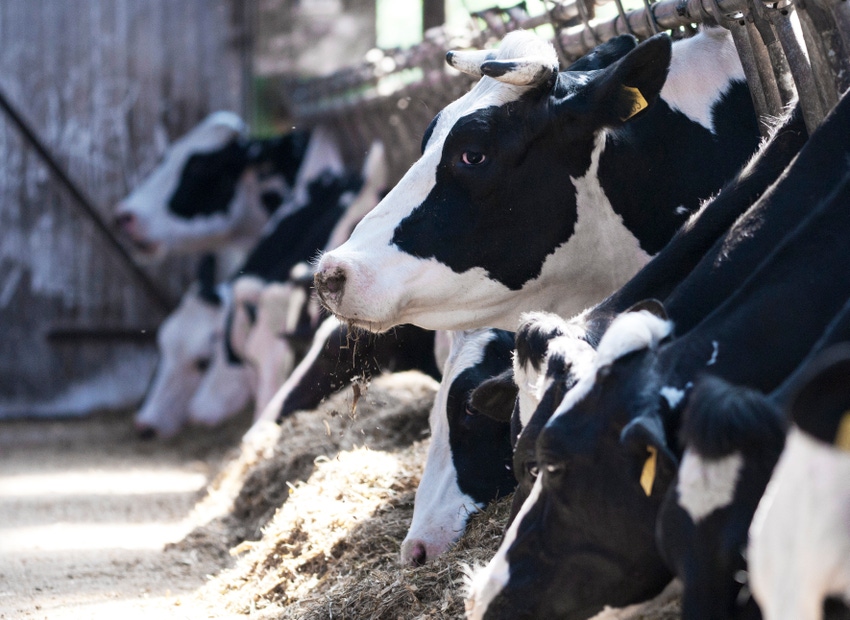
Work presented by Elizabeth A. Eckelkamp of the University of Tennessee during the American Dairy Science Assn.'s annual meeting assessed disease detection accuracy by precision dairy technology health alerts and machine-learning techniques. It was hypothesized that the technology would detect health events, machine learning would improve disease detection and the incorporation of cow history with behavior changes would improve disease detection.
Eckelkamp said the study occurred from October 2015 to October 2016 on four Kentucky dairy farms (1,374 cows). Health alerts were generated based on changes in eating (hours per day), lying (hours per day), standing (hours per day), walking (hours per day) and activity (steps per day).
The FREQ procedure of SAS 9.4 was used to identify true positives, true negatives, false positives and false negatives based on technology-generated cow alerts and recorded disease events by the lead researcher and dairy producers. Sensitivity, specificity, accuracy and balanced accuracy were also calculated. Daily information collected by the technology (eating time, lying time, standing time, walking time and activity) and cow history were incorporated into machine-learning prediction models.
The technology-generated health alert sensitivity remained between 13% and 48%, with a 91-97% specificity, Eckelkamp said. Maximum balanced accuracy achieved with technology-generated health alerts was 59% at the widest time windows of five days before to two days after and three days before to three days after the day of disease detection. The greatest balanced accuracy occurred when all behavior changes were considered in combination, predicting any possible disease instead of a specific disease.
All machine-learning analyses performed similarly and improved sensitivity and balanced accuracy compared with the technology-generated health alerts, Eckelkamp said. Sensitivity ranged from 67% to 90%, specificity from 42% to 87%, accuracy from 67% to 99% and balanced accuracy from 66% to 87% across time windows, disease categories and behavior combinations. Unlike the technology-generated alerts, machine-learning predictions were found to be best during the 24 hours before the day of disease identification and when individual diseases were predicted.
About the Author(s)
You May Also Like